Key Points
- Focus on the problem first and bound it to a system, even when developing a pilot.
- Use the right data, not lots of data. Domain expertise can help focus what data streams are critical and what are not.
- Don’t forget the user. Digital twins guide intervention to boost performance and need to communicate effectively and efficiently with the users.
- Think of the bigger picture. Develop a digital twin strategy for your organisation before all the pilots get out of control.
Problem, Plant, People and Data
Much has been written about the promise of digital twin technology and the benefit it brings to industry and the built environment. The foundations required to create an industrial utopia, void of inefficiency, are there, ready to go, but for some reason not everyone is embracing their twin as human siblings might. Perhaps the gap between the idea and the reality is bigger than expected, particularly when developing a digital twin for an existing building, machine or process. It’s easy to get distracted by the promises of machine learning or augmented reality but the key to success is understanding why a digital twin is needed, what it will be used for and who will use it. Data has a huge part to play but it’s a matter of proportionality, not quantity.
In this post we explore the key elements required to effectively develop digital twins, for industrial plants, such that operational performance can be improved and sustained over time. We focus our thoughts based on experiences and applications in the process industry and power generation sectors.
What’s your problem?
As with any emerging technology there is temptation to jump on the band wagon without fully reflecting on the need. Some organisations have invested in digital twin development only then to find out that the data isn’t there or that they’re not really sure to do with it. They have developed a nice toy but a toy doesn’t solve problems, it creates them. Understanding the core operational problems or opportunities is the critical first step in the digital twin development process. Digital twin development takes time and if the problem isn’t well defined then the outcome will be ineffective and underwhelming. This may cause the organisation to label digital twins with a warning, missing out on huge potential benefit in the future. The problem should be linked to a well-defined asset, system or process and should naturally present an opportunity for improvement. Ada Mode like to frame a digital twin project in three parts – the problem and system, the intention and the outcome. For example:
1 Problem and System: The maintenance of the turbine is extremely costly, and we often don’t find much wrong with it
2 Intention: A digital twin to assess and monitor turbine sub-system health will help us move to a condition-based maintenance strategy
3 Outcome: This will reduce our cost and increase output through reduced downtime.
System health is one example of the value a digital twin can bring to support wider business objectives, but it is important to consider the bigger picture before embarking on digital twin development. A sophisticated twin could provide health monitoring but also recommend control policy changes to improve process efficiency. Figure 1 shows the value hierarchy of digital twins in and industrial setting and how this relates to digital twin functionality.
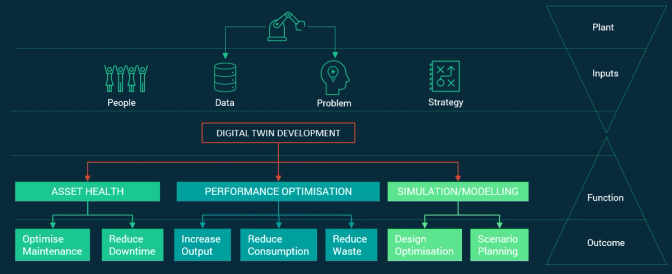
Once the problem has been identified its important to review the data landscape before diving into digital twin development.
Proportionality: The Right Data at the Right Time
The key to solving the problem or opportunity associated with the asset or system is the availability of the right data at the right time. It is important to understand the data landscape early on and ensure proportionality against the problem in question. For instance, it may be that a few data streams from a small number of subsystems are all that required to alleviate the pain. It may be the case that the problem is sufficiently complicated and painful that additional sensors need retrofitting, requiring greater investment. In all cases its critical to ensure domain experts and system owners are intimately involved rom the start of the process such that the development is targeted and optimised. A digital twin doesn’t need huge amounts of data, it needs the right data. The resolution of the data is also important and again needs to be proportional to the problem. A live data stream, sampled every second, may be appropriate for a process optimisation twin but is unlikely to be required for monitoring health.
One thing to think about is how will system requirements change over time. While you may not need every sensor feeding a data lake now, would it be useful to have that functionality for the future as the demands of the system change?
Its easy to think a digital twin to be an aggregation of different sensor feeds into one dashboard but the real power of digital twins comes when this sensor data is combined with wider contextual data. If there is a way to integrate plant walk-down inspections or historic maintenance inspections along with external weather data, for example, then this could lead to extremely powerful insights. Contextual data can help provide labels for more advanced functionality for supervised learning models to support event prediction and forecasting. A clear understanding of the problem and associated data will help to design the most appropriate analytics engine or machine learning models for the twin.
The management of data streams, particularly in larger disparate organisations, can be hugely complex especially if the data is sensitive requiring different layers of cyber security. Establishing a corporate digital twin strategy early on, before diving into development, will save time and money in the long term and enable wider benefit across the enterprise.
Who is using this technology?
Digital twins are required to provide insight or recommendations to facilitate intervention such that something can be improved. Some digital twins may have automatic links to a control system or a CMMS but the vast majority will be interfacing with human operators to drive the boost in efficiency. It is therefore critical that this interface is designed to ensure the best possible results, eliminating ambiguity, confusion and needless complexity. Not all digital twins need a translucent 3D CAD rendering. The person or people who will be responsible for using and managing the digital twin must be involved right from the start of the process to ensure success. In our experience the best results come from when the digital asset ownership mirrors the physical. That is to say the system owner responsible for operating or maintaining the plant also manages the asset’s twin. As such the user interface should be designed around their needs. If the same twin architecture is being used to inform management on general plant performance, then the interface design should adapt to reflect this – likely meaning a reduction in the level of detail.
Final Thoughts
The benefits from successful digital tin implementation are huge. Their use can drive down costs, increase safety, reduce emissions and increase output but it’s important to get the foundations in place before embarking on the journey. Make sure to understand the problem, plant, people and data first.
Over the next few months Ada Mode will be publishing a more detailed white paper guide on digital twin development for industrial plant. We are also hosting a webinar on the subject which will expand on this blog post. You can register here.
If you would like to hear more in the meantime, then please get in touch.