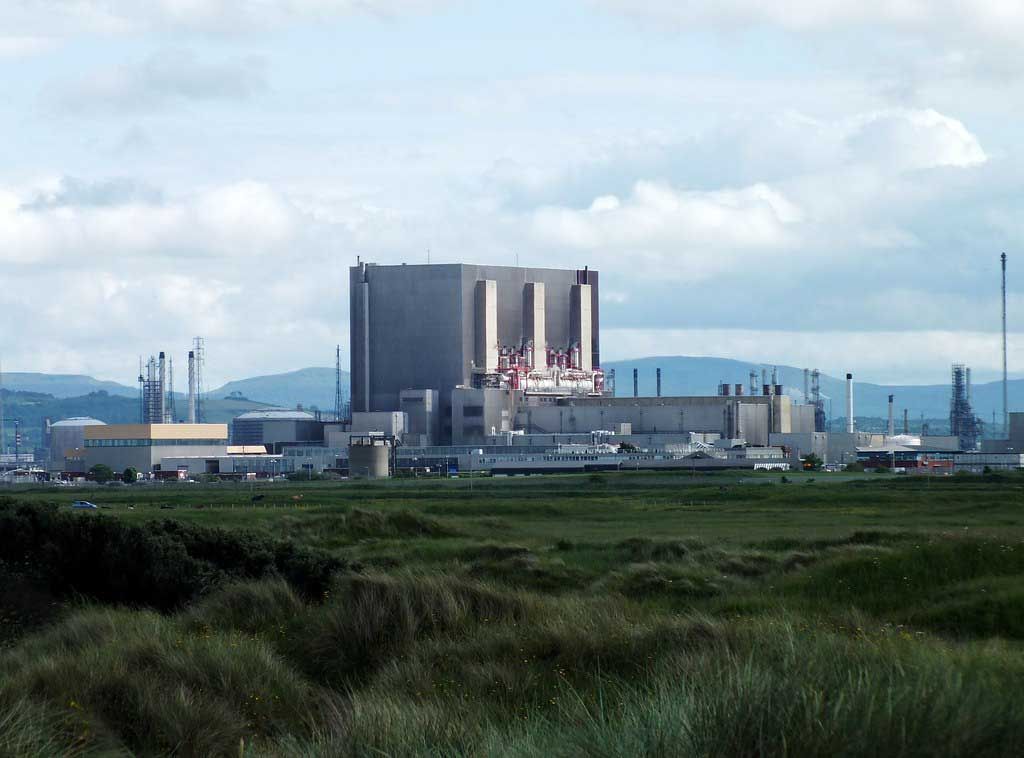
Intelligent Asset Management
Automatically achieve optimal process conditions to reduce waste and increase plant availability. Understand why machines are underperforming and what to do to restore productivity.
Case studyCustom applications built on Ada Mode’s industrial data architecture
Automatically achieve optimal process conditions to reduce waste and increase plant availability. Understand why machines are underperforming and what to do to restore productivity.
Case studyUnderstand where and when energy is being consumed. Reduce demand and consumption based on the optimisation of operational processes and energy availability. Reduce CO2 emissions and the cost of energy.
Case studyUnderstand the health of critical plant. Detect anomalous behaviour and direct intervention before failure. Eliminate unplanned downtime and reduce cost of maintenance.
Case studyCombine modern AI, physics modelling and operator experience to enable rapid deployment of powerful digital twin solutions at scale.
Secure cloud platform for customer data integration, model development and digital twin deployment.
Enables seamless integration of custom, human-in-the-loop digital twins within existing digital architectures and technology stacks.
Ability to integrate critical domain expertise and operator experience with outputs tailored to the user to guide intervention.
Atlas architecture is hosted on secure, carbon neutral AWS servers with the highest levels of security as standard.
Models and digital twins can be applied across a plant, region or enterprise at speed.
Developed to minimise the time from problem to solution without compromising on scale, analytical power, user experience or security.
Designed to accommodate a huge range of data sources for both live and batch processing. Data processing capability for time-series, unstructured text, numeric, graphical. GIS, video, audio and network data sets.
Cutting edge machine learning tools developed around complex industrial processes, systems and machinery.
Unsupervised AI technology for the detection of anomalous plant behaviour through live processing of disparate datasets including image, audio emissions, text and time series data. Developed to be non invasive and to be used on systems with limited or no failure history, it enables controlled intervention prior to a failure event of shutdown.
Supervised machine learning technology for the prediction and prevention of adverse plant conditions. Capable of multivariate analysis of diverse plant parameters for identification of associated factors enabling a more informed remedial design process. Incorporates trend detection and pattern matching technology for use on live data streams.
Technology to optimise a user defined output based on providing automatic recommendations to tune and change key process parameters. Used on complex process plants where multiple factors interact and where a detailed knowledge of plant systems is required. Increases output and enhances the human -machine interface experience.
A suite of tools used to support the day-to-day operation of industrial plant. Tools include trend detection, pattern matching, statistical analysis, forecasting and anomaly detection. Integrates with maintenance records and plant logs to provide contextual analysis. Enables users to interact with historic plant data to investigate current plant behaviour.
Not all industrial data is time-series or numeric. Our NLP technology is used to analyse unstructured text data found in plant logs, maintenance records and engineering reports enabling deeper contextual insight into plant performance. This technology is used frequently to optimise planned or preventative maintenance schedules based on mining historic inspection and overhaul data.
A framework for assessing system health and risk using a combination of AI and user defined criteria. This technology aggregates disparate plant data enabling operators to review plant performance in one place. Users are able to define health criteria such that when a breach occurs they can intervene in a controlled way. Operators can plan maintenance more effectively and monitor performance more closely.
Neural network powered AI systems for image recognition. Deployable on static images, video, and even audio data to perform highly accurate, scalable and automated image / audio classification. Powerful applications in a range of fields, including quality assurance, remote inspection, condition monitoring and acoustic leak detection.
An established toolbox of methods to clean, process, aggregate and analyse audio data. Wrangle audio data into formats more suitable to the application of data science techniques and extract key insights hidden from human observers, listen in on key assets and detect faulty operational acoustics.
Application of data science techniques and optimisation algorithms to explore simulations, physical and computational models. Develop a lightweight ML surrogate model of the simulation and use it to iterate through the design space in tandem with a multi-dimensional optimisation algorithm to finalise system or process design decisions.
Physical process models and simulations provide an additional baseline against which to compare target sensor readings. Alongside soft sensors, this approach can provide powerful insights into sensor health.